Machine learning in finance is revolutionizing the way companies in the sector operate. As a branch of artificial intelligence, this technology is driving financial innovation by optimizing processes, enhancing decision-making, and personalizing services. Below, we explore its benefits and how it is applied in real-world financial solutions.
Machine learning is a subfield of artificial intelligence. It refers to a system or program that trains a model using input data. Once trained, the model can make accurate predictions based on new, unseen data derived from the same patterns found in the training dataset.
What are the benefits of machine learning in finance?
- Increased operational agility: Automates repetitive tasks, reduces processing time, and improves overall business efficiency.
- Scalability: Allows financial systems to handle large volumes of data and users without compromising performance.
- Data-driven decision-making: Enables more accurate and comprehensive analysis, improving strategic outcomes.
- More effective regulatory compliance: Supports data verification, anomaly detection, and documentation of critical processes.
- Sustainable competitive advantage: Facilitates continuous innovation and better adaptation to change in dynamic markets.
Suscribe to our newsletter!
What are the applications of machine learning in finance?
Fraud prevention
Machine learning enables the real-time detection of unusual activity and suspicious patterns. Unlike fixed rule-based systems, ML models continuously adapt and learn. This reduces false positives and improves the accuracy of fraud detection. It also strengthens user security without disrupting the digital experience.
Credit scoring and risk assessment
ML algorithms analyze large volumes of both financial and non-financial data to estimate a customer’s likelihood of default. These models consider variables such as payment history, digital behavior, and contextual data. The result is more accurate credit scoring that expands access to credit without increasing risk.
Read more → QA testing: How to implement artificial intelligence and machine learning?
Personalization of financial services
By analyzing user behavior, preferences, and spending patterns, machine learning enables the delivery of personalized offers tailored to each customer. It goes beyond traditional segmentation to match financial products with dynamic customer profiles. This boosts retention, enhances satisfaction, and drives long-term loyalty.
Onboarding and automated support
Machine learning, combined with natural language processing (NLP) and computer vision techniques, is transforming the onboarding experience. Platforms can verify identities, analyze documents, and assist users through intelligent chatbots. All of this happens in just a few seconds, streamlining the process and reducing manual errors.
Document processing and text analytics
Automated text processing using trained models enables the analysis of large volumes of financial, legal, or regulatory documents. This streamlines tasks such as auditing, compliance, and document management. ML extracts and organizes key information quickly and accurately, significantly reducing operational workload.
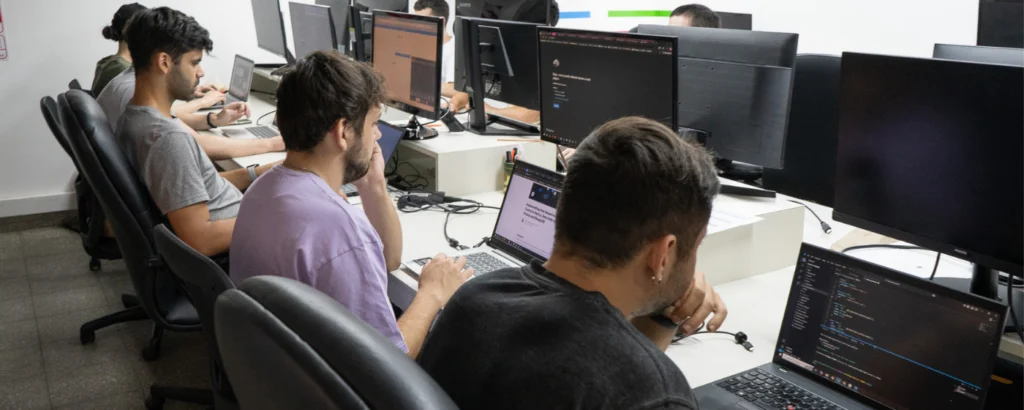
Automating compliance processes (Due Diligence)
Machine learning helps automate complex checks such as Know Your Customer (KYC) and Anti-Money Laundering (AML). By processing both structured and unstructured data, it accelerates background analysis and identity validation. This speeds up customer onboarding and strengthens regulatory compliance.
Predictive analytics and decision-making
ML models anticipate customer behavior, identify consumption patterns, and detect signals of risk or investment opportunities. This predictive capability supports proactive strategy development and informed decision-making. It offers key advantages in portfolio management, product design, and market analysis.
Smart payments and error detection
ML in payment systems enables the detection of duplicate transactions, identification of common errors, and prediction of payment behaviors. These capabilities reduce operational losses, increase system efficiency, and enhance traceability and control in complex payment environments.
Open finance and open data analytics
Machine learning makes it possible to fully leverage open data from the Open Finance ecosystem. By integrating diverse sources—such as banks, digital wallets, and fintech platforms—algorithms can generate more complete and accurate insights. This enables more inclusive financial products and personalized strategies for each user.
Read more → Discover how Crombie can help you enhance your operations with Open Banking and Open Finance
Tools for implementing machine learning in finance
Amazon Web Services (AWS) offers advanced solutions for implementing ML in the financial sector. Some of the most commonly used tools include:
- Amazon SageMaker: Trains and deploys scalable machine learning models.
- Amazon Rekognition: Enables identity verification through image and video analysis.
- Amazon Fraud Detector: Detects and prevents fraud in real time.
- Amazon Bedrock: Provides access to generative AI models for advanced financial applications.
These tools enable the development of secure, scalable solutions tailored to the needs of the financial industry.
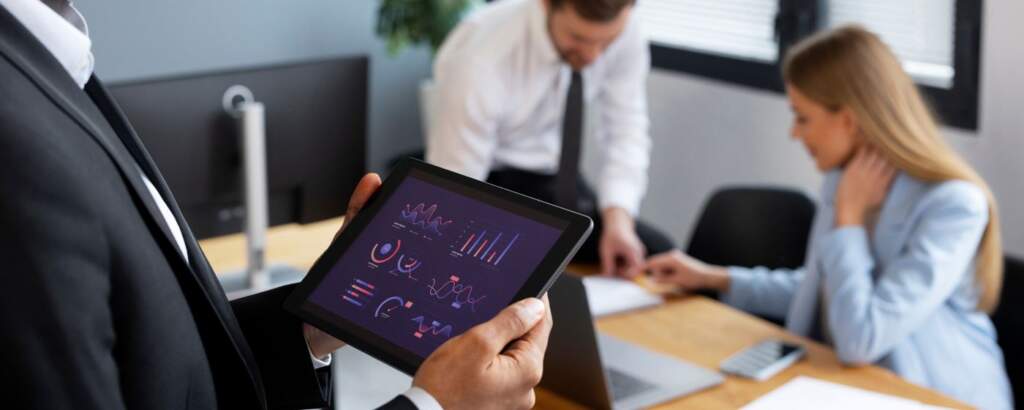
Conclusion: Why adopt machine learning in finance?
Machine learning in finance doesn’t just improve internal processes—it redefines how companies interact with users. When implemented strategically, it enables innovation, reduces risk, and improves responsiveness to market changes.
Companies that incorporate this technology gain a real competitive edge. At Crombie, we integrate artificial intelligence and machine learning into our fintech solutions to scale operations, automate critical processes, and deliver high-impact financial experiences.
Want to optimize your financial operations with artificial intelligence?

FAQs
It’s the use of machine learning algorithms to automate, optimize, and personalize financial services.
The future of ML in finance lies in broader and more strategic adoption. As models become more accurate and accessible, institutions will be able to automate complex tasks, deliver hyper-personalized products, and better anticipate market risks. Combined with technologies like generative AI and real-time analytics, ML will continue to drive innovation and efficiency in the financial sector.
Leave a Comment